The goal of this project is to develop a failure detection, isolation and recovery algorithm (FDIR) for a cubesat, but using machine learning and neural networks instead of the more traditional methods.
Motivation: What is an FDIR algorithm and why is it usefull?
One of the most challenging parts of space missions is knowing and controlling where your spacecraft is, what is its relative orientation with respect to earth and how it is moving. Being aware of these three things is crucial to know if your spacecraft is flying too high or too low, too close to other spacecrafts, or simply if its oriented in a way that will allow it expose its solar panels to the sun to produce power or to point its antenna down to earth for calling home.
To perform this crucial task of computing and controlling its position and orientation spacecraft are designed with a variety of sensors and actuators that, together with proper control algorithms, ensure that your satellite remains where you want it and pointing in the right direction. This is often referred to as Attitude and Orbit control subsystem or AOCS.
Since this subsystem is critical for the spacecraft, it is needless to say that a failure in one of these sensors or actuators could easily kill your spacecraft and put and end to your mission. A faulty reading of a gyroscope that says that your spacecraft is rotating when it is not, a dead gyroscope that gives no signal and can’t tell if it is, or just a faulty thruster that provided more thrust than it should could leave your spacecraft spinning uncontrolled.
For these reason, providing the spacecraft on board software with a way of detecting these kind of failures as well as guidelines on how to proceed if one of these failures is detected is crucial for any space mission. This is done by means of the so called Failure Detection, Isolation and Recovery algorithms (FDIR).
Traditionally, these types of algorithms where simple, as they where based mainly on hardware redundancy , i.e., having many sensors that measure the same thing so that if one fails, you could detect the failure by looking at the rest and seeing that the signal is not consistent, isolate the failure by ignoring the reading from that sensor, and recover from the failure simply by continue to listen to the rest non-faulty sensors. While this is a valid and robust strategy to FDIR, it requires hardware redundancy of many spacecraft sensors and actuators, which means carrying on board more gyroscopes or reaction wheels than you actually need.
In recent years however, there has been a rising interest in low-cost space platforms such as Cubesats, pico or nano satellites that perform missions with much smaller budgets. One of the strategies used in these missions to reduce costs is to replace hardware based functionalities by software based ones, reducing therefore the number of components on board, the weight and power demands and overall complexity.
Replacing a hardware redundancy based FDIR strategy with a software based strategy is a perfect example of this. If your on board computer is capable of detecting a drift or a bias in the measurement of a sensor and correcting it without the need of comparing it with redundant sensors, or comparing it with the smallest number of redundant sensors possible then your mission might still be capable of safe operation, but minimizing the weight, power and cost penalties of hardware redundancy. There many ways to perform FDIR algorithms that focus on software instead of hardware, in order to explore some of the less conventional ones, it was decided to focus the project around machine learning and neural networks.
Project description
The goal of the project was then to set the basis of a neural network that could work to detect possible faulty signals from a cubestas sensors and actuators during its operation. This project had then two distinct lines of work:
- To develop or modify an existing simulator of a Cubesat to generate and extract the data from the sensors and actuators. This data is needed to train and test the Neural Network.
- To create an script capable reading and preprocessing the data from the simulator as well as creating, training and testing the neural network.
For the first, task an existing Cubesat simulator that included its own FDIR algorithm was used. This simulator written by Javier Sanz Lobo using Simulink included among its features the ability to simulate not only the cubesats motion, but also the readings from gyroscopes, reaction wheels and thrusters, as well as the capacity to induce artificial failures on the different components during the simulation. The original simulator can be found linked in the following repository:
https://github.com/msanrivo/SmallSat_FDIR
Several modifications where made to this simulator in order to fit it to it’s desired purpose. Among these it is worth highlihting:
- The ability to Change the initial conditions to randomly generated ones within a desired range
- A Matlab code that ran the simulator in a loop with random failure scenarios to generate the training and testing data
- The capacity to export the readings from the sensors and actuators during the simulation to .txt files with the desired format.
- Disabling the FDIR algorithm so that the simulated readings of the artificial failures remained unchanged.
- The capacity of failures to occur at different times rather than at the start of the simulation.
For the second line of work, a scrip was written from scratch in python 3.8 using keras from the TensorFlow library to build, train and test the neural network. At the day of publishing this post, there are currently two scripts that read the data from 6 gyroscopes and 4 reaction wheels of the cubesat in the simulator and use one thousand simulations to train a Neural Network and a convolutional neural network. In both cases the network is then tested with another one hundred simulations to evaluate its real accuracy.
The end result is two types of neural networks that are both capable of predicting not only the most likely failure scenario that corresponds to that data, but also the probability of each individual failure scenario, which is a valuable input for future steps in the process of developing a fully functional FDIR algorithm.
Results
Currently both the traditional neural network and the convolutional one achieve accuracy values of around 70% in their predictions. Note that with 6 gyros and 4 Reaction wheels and the limitation of a maximum of two gyros and two reaction wheels failing the number of possible scenarios rises up to 242, which makes it hard to perform predictions.
Simpler scenarios where none of the devices fail are easily identified by both networks, as is the case of the following figure where both predict the correct outcome with a probability higher than 70%:
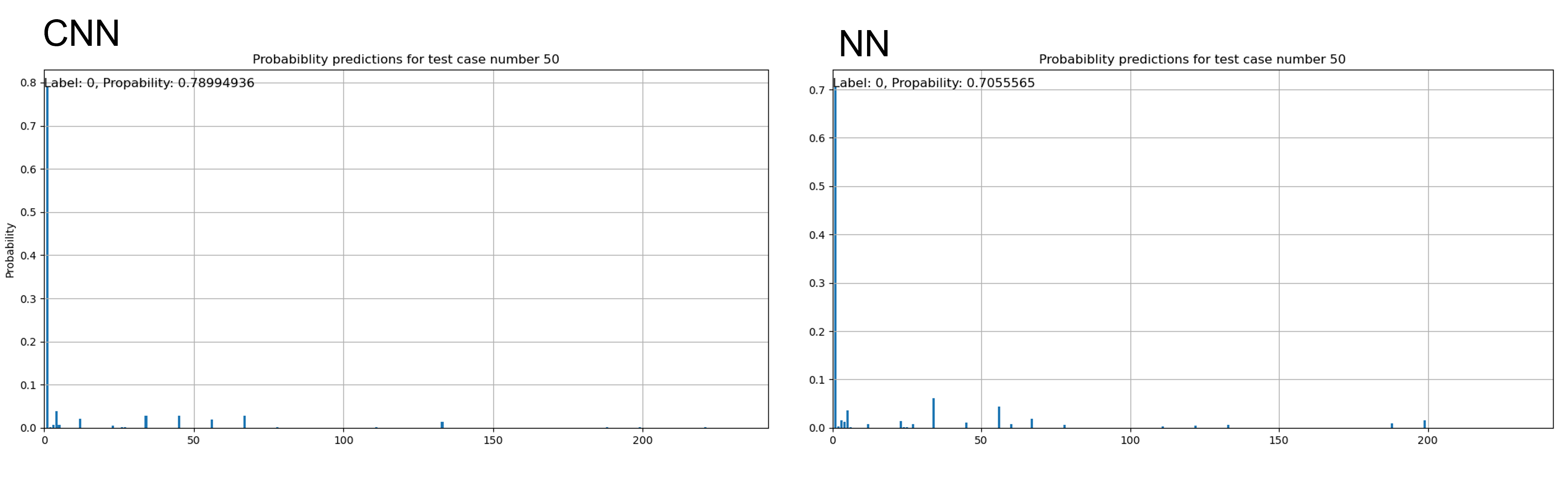
More complex scenarios where one or several devices fail reduce this prediction probability, making them capable of predicting correctly the failure of one device, but rarely all of them. In this cases, however, usefull information is provided by the probabilities, as the correct scenario can be found among those with the highest probabilities even if it is not the one with the highest.
Take for example the case depicted in the following figure where only one reaction wheel fails. The CNN is capable of predicting the correct scenario, but the NN predicts a scenario in which not only the aforementioned wheel fails, but also two complementary gyros as well. Note that even when predicting the wrong scenario, the NN shows the correct one as the second most likely.
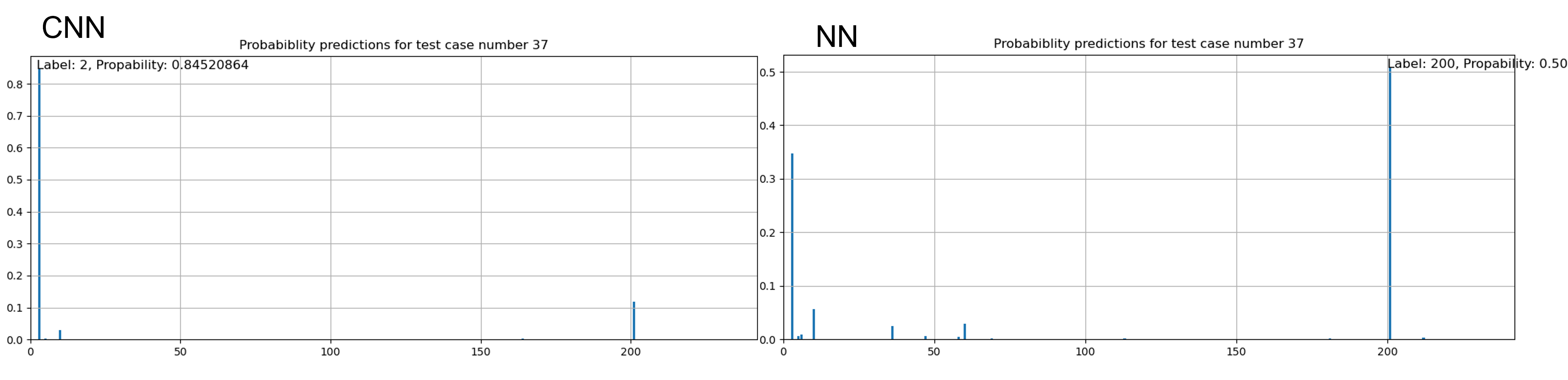
Future work
A lot has been achieved during this GSoC period, yet there is still plenty of work ahead in this ambitious project. Among the features that are still to be implemented and tasks to be performed there are:
- Continue to improve the neural networks to achieve better results at higher computational efficiencies.
- Improve the simulator to generate better failure scenarios for thrusters.
- Expand the FDIR capabilities of the network to include the simulated values of thrusters.
- Research on reducing the number of input arguments to reduce the order of the problem and increase efficiency.
- An interface between simulator and neural network to test and simulate in parallel.
Useful links
Github Repository: https://github.com/Rafabadell/FDIR_Neural_Networks
Author e-mail: Rafael.Badell@aerospaceresearch.net